Melissa Gander, chief operating officer at Kaluza, runs the rule over a number of modern customer support operating models to help guide energy companies towards improved decision making, innovation and service.
Never before has support for energy customers been so critical to get right.
So how can energy companies organise their support centres to deliver outstanding service, now and in the future? What are their options as they look to evolve their operating models? These questions are being asked by many of our clients at Kaluza.
Technology is key
Across energy retail and beyond, we’re seeing a growing trend of companies moving towards more agile, cross-functional operating models. What has typically been a set up for technology and product teams is now being adopted across customer support operations.
This is a fascinating shift which I’ve observed for over 15 years in energy, mostly spent leading operational teams of varying sizes and types – from customer service through to field engineering – for Ovo Energy, now the UK’s third largest energy supplier. I’ve seen, first-hand, Ovo’s customer operation grow from a handful of people in a barn in the Cotswolds, to thousands of advisors in a combined operating model with SSE.
What has become clear in this journey is that the strength of the technology in use is critical to the success or limitations of each operating model. For example, an early attempt at moving to the ‘squad model’ in 2013 was a great example of when technology didn’t support the model and led to the change being scrapped at the pilot stage. This is the reason I joined Kaluza – to build technology that enables retailers to transform their operating models and in turn deliver the services we need to decarbonise homes.
The ‘traditional’ model
The typical customer support operating model has evolved to become heavily segmented.
First are front line teams split by core skill set, then sits a second line of more experienced advisors who are further segmented into specialisms to manage more complex cases, and ultimately ‘back office’ teams who fix even more complex issues.
Altogether, issues are mainly handled reactively following customer contact although there is some proactive resolution across the segmented teams.
A typical set up looks like this:
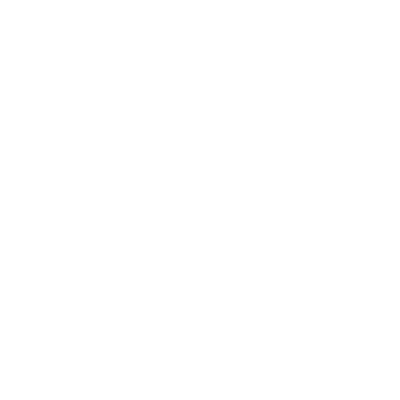
Why is the model so heavily segmented? Most probably due to the level of system-driven complexity faced by advisors in completing key processes.
Front line advisors are trained to handle a relatively small range of short, transactional tasks with limited system access, while second line support have deeper training on specific specialisms and enhanced access enabling them to fix underlying issues.
Segmenting teams in this way reduces the volume and diversity of tasks an individual advisor needs to learn and be proficient at on the system. It’s based on traditional retailers’ focus on output over outcome, where speed is the priority.
The theory is that advisors will always be faster, and more accurate, when repeating one process rather than switching between 10 or more processes.
However, this operational approach only accommodates the fundamental challenge of high system complexity. This complexity is the result of a number of different factors. For example, user interfaces and processes have been so tightly coupled with the ‘back end’ system that process flows mirror the data architecture beneath the surface – advisors practically see the architecture of data tables and have to navigate multiple screens at multiple levels to complete simple tasks.
Additionally, numerous workaround processes, ‘bolt on’ widgets and shadow IT are added over time to compensate for deficiencies within core systems.
The end result? Multiple handoffs per query – from front line to second line to back office teams.
Each step puts a customer issue back into another queue for another advisor to pick up and attempt to solve. This leads to workflow backlogs, long customer wait times and non-customer facing teams handling issues, culminating in a dissatisfying and disjointed customer experience.
Advisors’ morale also takes a hit, with many becoming frustrated by their inability to provide better service due to their limited training and access. Team and individual goals are often unaligned resulting in siloed ways of working and a lack of accountability, causing further anguish and disengagement.
Suddenly, the benefits in individual efficiency are reversed as resources are pooled (think Erlang C) and bigger issues arise in the form of decreased resource resiliency and flexibility.
In summary, poor, patched-up tech has necessitated moving to increasingly complex operating models – negatively impacting efficiency, flexibility, advisor happiness and, most importantly, customer experience.
The new ‘squad model’
Spotify claims the title of creator of the ‘squad model’ whereby product people are divided into very small teams, known as ‘squads’, each owning a certain part of functionality end to end, such as ‘search’ or ‘recommended artists’.
A squad will have a dedicated product manager who will work with other skilled members in their team to solve problems in the best way possible for a given customer journey. These squads sit together and have one long-term mission. They are an autonomous, self-organising unit possessing all the skills, tools and expertise needed to design, develop, test and release to production – comparable to mini start-ups.
So how does this default model for software development translate into an operational environment?
The emerging approach is to set up a cross-functional team made up of multiple skill sets, for example, front line care advisors, back office experts with individual specialisms, complaint management experts etc. and allocate to them a cohort of customers to manage. The team is headed up by a squad leader whose role is to oversee the management of their customer base to drive key business metrics.
The squad leader can choose where to focus their team – from proactive work to reactive work; from focusing on commercial metrics to service metrics – they make the trade-offs.
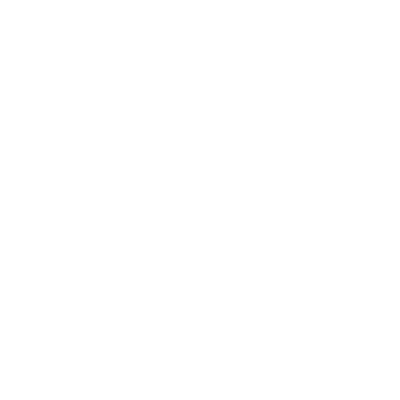
An example could be choosing to allocate more team members to outbound customer loss prevention activity rather than inbound call answering – leading to higher wait times and theoretically lower customer satisfaction but increasing customer retention. Team members still report into their functional ‘homes’ – care advisors into care leadership, back office specialists into their respective specialist leaders.
The squad model has many advantages:
- End-to-end ownership of a subset of customers increases accountability and ownership. Individual team performance can be tracked against customer outcomes; something not possible in the traditional model.
- Customer satisfaction can be dramatically increased as issues are dealt with end to end by the same individual. Experts are immediately available who have all the context and information about that specific account. Allows for rapport to be built with the customer and a more seamless experience.
- Issues can be resolved faster with fewer hand-offs as a multi-skilled team handle requests who possess the full set of capabilities needed to resolve issues reducing the need for multiple hand-offs.
- Team mentality is strengthened as each team is accountable for their own set of customers in contrast to a large contact centre environment. Working on a greater variety of tasks creates higher job satisfaction and advisors are generally more motivated to deliver quality service, and prevent issues resurfacing for another squad member to handle.
- Enables a shift to proactive customer service as improving first contact resolutions allows teams to spend time reactively supporting customers or proactively identifying and fixing issues before they reach customers. The more issues are fixed proactively, the less reactive teams need to be, resulting in vastly reduced inbound customer contact.
The model supports Daniel Pink’s motivational model, with mastery, purpose and autonomy as the drivers of motivation and productivity:
- Autonomy – Advisors and their teams are empowered to identify how best to service their customer base in balance with commercial outcomes and how to structure and prioritise their time.
- Mastery – Advisors don’t have the constraints of a heavily segmented model putting boundaries on the scope of their learning. Advisors develop each other through cross fertilisation of knowledge and best practice from the deep-narrow skilled advisors to the broader-shallower skilled advisors, and vice-versa.
- Purpose – Teams can see the direct results of their actions through the performance metrics for their subset of customers. So productivity, retention and engagement increases – leading to reduction in the cost of underperformance, attrition and absenteeism.
It is early in the migration to this way of working for energy retail and so it is potentially premature to opine on its success or limitations. I see the following challenges that should be considered when designing the model:
- Significant and simultaneous change across process, culture and mindset. Treating the transformation as a ‘care+’ model, whereby second line or back office teams are allocated into an existing Care structure, risks failing to truly transition away from existing reactive, rules-based practices. Cultures, ways of working and often leaders need to change in order to make the transition successful. What aspects of operations are kept outside of the squad model, in ring-fenced functions, also needs to be considered.
- Small teams reduce flexibility and resilience. As the traditional model grapples with inefficiencies caused by segmentation, loss of large resource pools; the same is true of the squad model, having small teams reduces the pool of advisors ready to support a customer at a given time. It also reduces resilience, when a skill set is less available within a team, due to absence or attrition, backlogs form far faster than they would with larger resource pools absorbing the work.
- A great team leader doesn’t necessarily migrate to a strong squad leader. Rather than a traditional team leader who focuses on people performance; a squad leader is focused on the performance metrics for their customer base, including commercial outcomes.
- Overqualified front line advisors. As the model is dependent on having highly skilled front line advisors, it might not suit organisations that have a high volume of low complexity calls. Advisors able to handle the most complex queries might spend the majority of their time on basic transactions that could be supported by lower skilled, lower paid advisors.
- Outsourcing becomes difficult. Many businesses now outsource high volume, low complexity work. The squad model depends on having highly autonomous teams and individuals whereas a typical outsourcing relationship lends itself to clearly defined parameters and processes. Will outsourcers struggle to work with the autonomy and flexibility of the squad model?
- Risks of unclear accountability. Where does the ultimate accountability for business metrics sit? For example, is customer retention purely a squad metric or one owned by commercial teams? If both, how does the relationship work between a centralised commercial team and a distributed set of squads? That might also be the case for operational metrics like debt recovery or billing performance. Careful thought is needed to ensure cross-functional working is effective and all teams are aligned; otherwise core metrics could be unowned or be pulled in conflicting directions.
- Specialist knowledge could be diluted. Team members report into their functional ‘homes’, care advisors into care leadership, back office specialists into their respective specialist leaders. Ensuring true cross functional reporting lines is key to retaining specialisms and preventing the specialist knowledge and skill sets being neglected.
- Data is critical. Without access to the right data to support performance tracking, proactive working and resourcing then the model will fail to deliver the perceived benefits. To set the right practices, behaviours and culture, one would argue that this data-led approach should be implemented from day one, adopting an approach of adding it ‘later’ risks undermining the very principles of the model.
On balance, while there are many advantages to the squad model, there are also some clear risks that spark scepticism and nervousness amongst retailers.
Is there an alternative approach?
After evaluating these two models, energy companies face a dilemma.
Sticking with a segmented traditional model prevents them from delivering a seamless and effective service for customers while the newer squad model requires a complete and complex overhaul of their current operations creating significant risks along the way.
I think there is room for a hybrid approach that retailers can take in order to reap some of the rewards of a less heavily segmented model without having to rebuild from scratch.
In this model, advisors fall into two categories; generalists or specialists. Generalists handle high-volume, low/medium-complexity queries and specialists handle low-volume, high-complexity queries, whilst also proactively resolving exceptions.
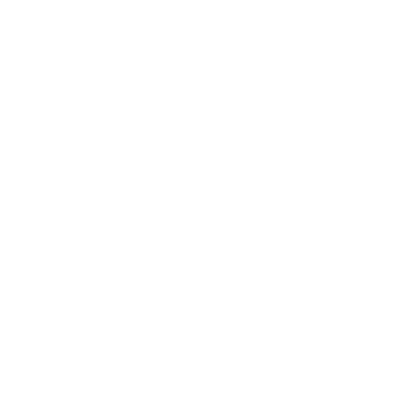
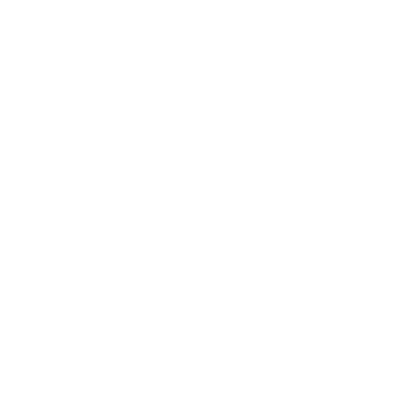
This simplified model is similar to the squad model in that there are generalist and specialist skill sets, however, customers aren’t allocated to small teams.
Whether this model succeeds comes down to three main factors:
- Finding the most effective ratios of generalist to specialist skill sets which is unique to that retailer
- Ensuring that the specialists are adequately resourced to continue to react to inbound queries while transitioning to a more proactive way of working
- Implementing the right technology to maximise efficiency
The role of technology
Technology has the power to simplify customer support operations, we have seen this as companies move away from the system-flawed traditional model.
The latest platforms are making advisors’ interfaces simple and intuitive, for example, by reducing the number of times they have to click to service a request from 27 to four, based on Kaluza data.
Speed to competency dramatically falls as advisors are better equipped, and repeat contact, failure demand, from customers reduces.
This has a few implications, regardless of operating model:
- A vastly-reduced requirement for ‘second line’ skill sets. Advisors are given the tools they need to solve customer problems at the point of contact
- Fewer queues and backlogs of work to be done
- The reduction in queued work means resource is freed up to proactively tackle exceptions before customers are aware of them
In an exciting advancement, we are also seeing the development of AI tools able to proactively flag anomalies in customer accounts, support diagnosis and provide advisors with recommended next steps.
What does this mean for which operating model to choose? While changing operating models has inherent benefits, deploying the latest software means operating models can naturally evolve to become less segmented and more efficient.
For example, by leveraging advanced analytics, technologies can maximise the value of every contact that is received. First, by enabling agents to fix issues faster and more effectively at the first point of contact and second, by using the conversation to add value, whether flagging an overdue payment or promoting a new product.
In addition, surfacing information about the customer allows the advisor to provide a personalised experience to enhance customer loyalty.
Further, platforms that are powered by live data can automatically generate workflow items for issue resolution, use customer behaviour predictive analytics and accurately forecast reactive vs proactive resourcing requirements. The more advanced the data capabilities are, the more proactive an organisation can be.
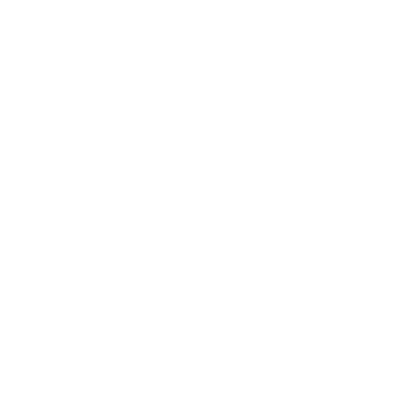
Decision time
So, which is the better model? The answer depends on the metrics a retailer is optimising for.
With an absolute focus on reducing cost-to-serve, one could argue that the optimum model is one with pooled, lower skilled advisors on the front line being supported by a more experienced layer of higher skilled, segmented reactive and proactive advisors, not aligned to a specific cohort of customers.
If optimising for customer satisfaction, the squad model with its end-to-end ownership of issues will likely yield better results. If gross margin enhancement through cross sell and upsell is the ultimate aim, then there are pros and cons to each – the squad model would lend itself to this more naturally as teams can own a P&L; although, sales is a specialism so the segmented model might lead to higher conversion rates.
However, important to any type of model is facilitating proactivity – actively looking for issues and resolving them before they manifest. Yes, it might seem cheaper to respond to a problem only when a customer flags it, but in most scenarios the issue has a customer and a business impact. Fixing an issue sooner will reduce the impact, improve customer experience and remove the cost of handling an unnecessary call. Moving towards a more proactive model will help achieve these results and deploying the right technology will multiply them no end.
Of course, the transition from reactive to proactive model is not easy. It entails considerable cultural change that requires careful planning and execution but, depending on which model is selected, the shift doesn’t have to require wholescale transformation. Ultimately, this evolution is an essential part of future-proofing the energy retail business and enabling companies to deliver the quality of service customers need, especially in these challenging times.
In summary, there are a multitude of options available to retailers both in terms of established models and new variations, implementing the ‘right’ one depends on the desired business outcomes. The effectiveness of all of these models can be supercharged by adopting emerging technologies and in many ways, deploying the best technology can reshape operating models organically.
See this content brought to life at Utility Week Live, 17-18 May 2022 NEC Birmingham. FREE to attend for utilities. Register today
Delivering best in class customer services is one of the frontline challenges at the heart of Utility Week Live 2022’s live content programme. View the programme.