Standard content for Members only
To continue reading this article, please login to your Utility Week account, Start 14 day trial or Become a member.
If your organisation already has a corporate membership and you haven’t activated it simply follow the register link below. Check here.
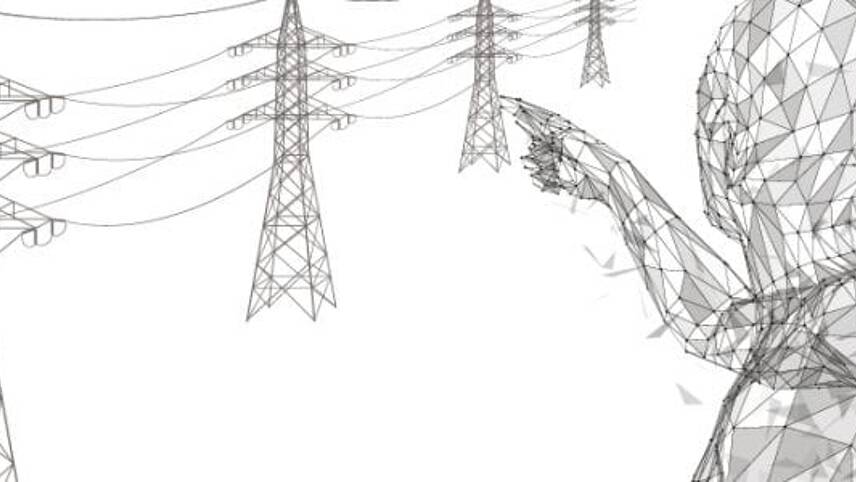
Machine learning is at the heart of a smart grid and future localised energy system
The art of balancing supply and demand on the grid used to be a relatively straightforward affair.
In days of old, the grid was awash with fixed assets, such as coal-fired power stations, which could be relied upon to generate a constant stream of power, night and day.
But now the UK has a more complicated energy ecosystem, with more assets to manage and a greater share of renewable energy.
And the advent of electric vehicles (EVs), localised grids such as Moixa’s recently announced virtual power plant in Sussex, and home energy trading platforms such as the one developed by Social Energy, all point to a world in which the manual balancing of the grid will become increasingly difficult, if not impossible.
For many in the energy sector, the answer lies in artificial intelligence (AI) and other machine learning systems to predict what energy will be needed and how best to supply it.
Speaking to Flex, the National Grid Electricity System Operator’s (ESO) energy intelligence manager, James Kelloway, says machine learning will be integrated into “many aspects” of the power grid and its control systems “in the not-so-distant future”.
“Machine learning is exceptional at spotting patterns that are not always obvious to even the most talented people,” explains Kelloway.
“It can predict ahead quickly, accurately and adjust automatically to changes in the grid and the external conditions that influence its operation.
“Decentralisation results in a great increase in data and consequently there needs to be a much larger capacity to deal with that data and associated actions and patterns. For context, Germany went from 1,000 generators in 2000 to an estimated 1.5 million not long ago.”
Kelloway adds that the core machine learning work at the ESO is currently all based around forecasting.
“Solar forecasting has been machine learning driven since last year and we are in the process of standing up enhanced forecasting capability for all other aspects of demand to enable a more efficient integration of renewables on the system to minimise consumer cost and carbon dioxide emissions from the generation fleet.”
Making AI while the sun shines

Another example of how the sector is working to create a smarter grid can be seen in the recent announcement that EDF Energy and its technology partner Upside Energy have signed a deal to optimise a combined 16MW of solar and battery assets at Clayhill solar farm in Bedfordshire.
Under the agreement, algorithms and artificial intelligence systems developed by Upside Energy will be used to analyse all aspects of the revenues available to the site and understand how to precisely optimise the assets to their full capability.
“We see in the UK that there is an appetite for this kind of solution,” explains EDF Energy’s director of energy solutions, Vincent de Rul.
“It is necessary from a strategic point of view, particularly when you want to push low-carbon solutions,” he adds. “It also has the capability to bring back the stability of the grid and integrate more renewables on the grid.”
EVs and grid management
The other major factor in the shift to a smarter grid is the transition to EVs. Although opinions are divided about when the shift from petrol to EVs will occur, there is no doubt that it will have a huge effect on grid management.
In particular, Kelloway says machine learning could also help in predicting the demand for charging.
“If the machine learning is aware of bus timetables and traffic conditions, this may assist in being super-efficient at predicting the associated charging demand,” he adds.
Using real-time weather data to forecast electricity with AI-driven systems will also play an increasingly large role in the grid of tomorrow, says Kevan Mossman, transformation director, part of the Odgers Interim network.
“It will also enable them to incorporate decentralised energy generation and battery technology into the grid and anticipate how this will impact demand,” says Mossman.
“At the same time, AI can run real-time analysis of a company’s grid and provide a working model of whereand when parts of it are likelyto be impacted by natural disasters or peaks in consumer behaviour.
“For example, it could tell you when a storm is due, the catchments it will hit, which pumps will be affected and whether there are any other parts of the grid that can be made available. This will enable companies to manage energy costs through spot price management.”
The concept of microgrids and virtual power plants is still in its infancy, but this is another area in which AI will play a leading part in managing supply and demand, as well as empowering local residents to trade their unwanted electricity.
In April, Moixa announced plans to create a virtual power plant in Sussex, as part of a scheme that could potentially save the country up to £32 billion.
Moixa’s GridShare platform will aggregate more than 1MW of spare capacity from batteries in homes, schools and council offices, providing a range of services to National Grid, energy companies and energy distribution networks.
The platform will use machine learning and artificial intelligence to tailor its performance to customers’ needs and maximise their savings, and this is expected to cut home energy bills by up to 40 per cent.
AI has its limitations
But Mossman warns that these systems will require large data sets to be fed through machine learning systems – something he adds that utility companies have historically been hesitant about introducing for fear of “losing control”.
Rob Richardson, who heads up the data science work at Habitat Energy, which has developed an optimisation and trading platform for grid-scale battery storage, adds that data inefficiency is a big limiting factor in how AI will be used to develop a smart grid.
“In principle, AI can solve any problem, provided it’s fed enough data and given enough time to train and interact with a real-world environment. However, these are major caveats,” adds Richardson.
The chief executive of Upside Energy, Devrim Celal, believes the availability of more “granular” – or detailed – data is necessary in order to prevent “issues before they happen”.
“You can understand how better to invest money into the right places, so you are building resilience in the right places. And when things fail, you can recover from those failures much quicker,” he explains.
“One of the projects we are looking at at the moment is collecting data from lots of different distributed energy resources – batteries, solar PV [photovoltaics], etc,” he adds.
“Each one of these assets would have a different way of behaving. If you start developing deep knowledge about their behaviour, you can monitor them and pick up any change in behaviour, as a means of identifying cyber threats early on.”
A question of cyber security
There is no question that a more automated and smarter grid raises important questions around cyber security. The Boston Consulting Group and the World Economic Forum recently published a report on cyber resilience in the electricity ecosystem, which called on utility companies to take more preventative action to protect energy supplies.
According to the Boston Consulting Group’s head of cyber security practice, Walter Bohmayr, there are now “constant attacks” on power grids everywhere around the world.
“There are computer systems out there that do nothing else but frequently check the security of utilities and try to find vulnerabilities,” Bohmayr explains.
“Attackers try to send false signals to the AI-supported defence systems and lure them into the wrong directions.
“They can falsely train the defence mechanisms and then get an advantage, and then launch an attack which gets overlooked by the defence mechanism.”
But the chief executive and founder of smart hub firm Verv, Peter Davies, believes energy systems are no more at risk than other sectors.
“If I was a hacker I would far rather go after the banks than the energy companies,” says Davies.
“The key thing with the grid is always security of supply. The consumer wants to know that electricity will constantly come to their home. They want to know that no one is doing anything risky with the grid that might possibly stop that. And on the flip side, if they believe that the use of data will lead to a better service and keep it more secure, then I think they will be in favour of it.”
The direction of travel does seem weighted in favour of AI. In July last year, predictive field service management company Oneserve commissioned research that found a quarter of utility companies in the UK have integrated AI into their systems. A further 37 per cent have plans to follow suit in the next five years.
And as smart and interconnected technology plays an even bigger part in our everyday lives, and the grid becomes ever more complicated, AI will become the norm. Many grid systems already run on a semi-automated basis with manual overrides – so perhaps a fully automated grid is not that far away. In the grid of tomorrow, data could be just as important a commodity as energy itself.
Power to the people

An automated and smart grid is good news for consumers looking to reduce their bills, according to Moixa Technology’s chief technology officer, Chris Wright.
The British smart energy firm has been working with the Japanese trading house ITOCHU to provide the AI ‘brains’ behind its 10kWh SmartStar Energy Storage System (ESS).
The ITOCHU system uses Moixa’s GridShare Client machine learning service to understand how the storage system is operating and provide customers with live data and regular feedback on their energy flow and savings.
Wright says 1,300 to 1,500 of these storage systems are now being rolled out in Japanese homes every month.
“We are seeing the cost of energy decrease by significant amounts,” he explains. “On average, we are showing that we can achieve a reduction of between 40 and 50 per cent compared to the standard behaviour of the ESS as our Japanese customers come out of the feed-in tariff.
“By predicting how those homes will consume energy for the next day, you can plan ahead. For example, you could make that home available for a flexibility service in the afternoon, when usage is low.
“We use machine learning to learn about customers one by one,” adds Wright. “So, while some energy companies might use average statistics to predict activity, we collect consumption data from homes every 30 seconds. We understand how they use energy and we understand how they generate energy.
“Then we can take that information and combine it with weather forecasting data. We then apply other algorithms and generate a prediction of what that household will do over the next 36 hours. We can then generate an optimum plan for that household over that time period.
“The AI is standing in the place of the consumer. This technology is working for the consumer, without them having to make any interventions.”
This article first appeared in Flex, issue 3. Read the full issue of Flex here
Please login or Register to leave a comment.